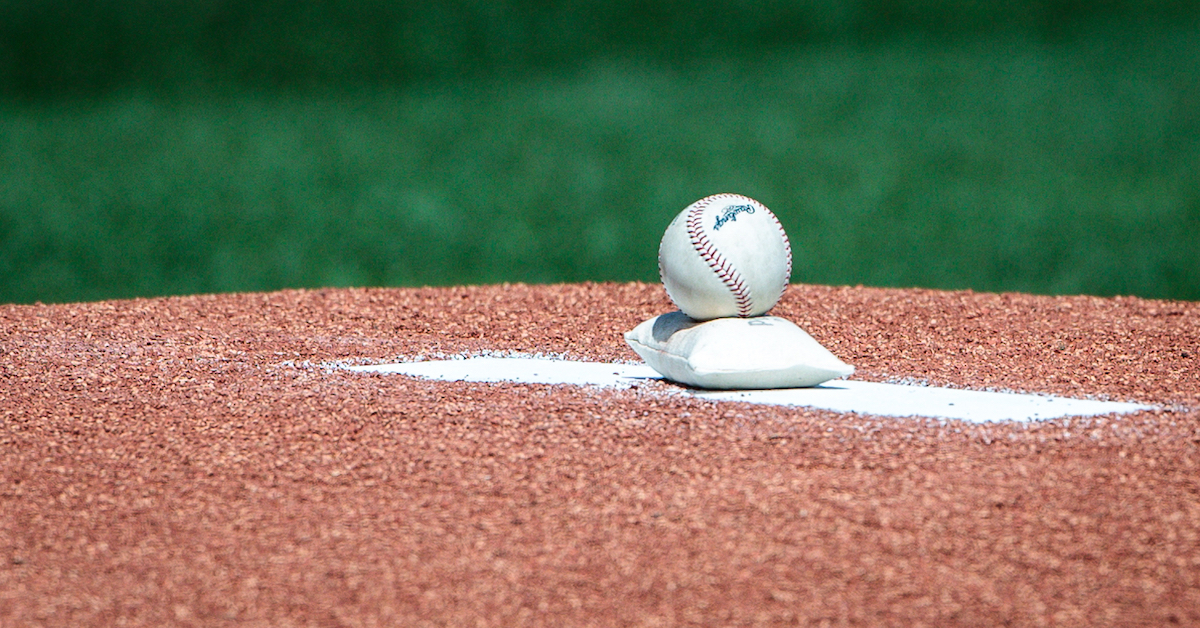
Love, Demise, and Pitching Robots, Pt. 2: Coming to Grips with New Know-how
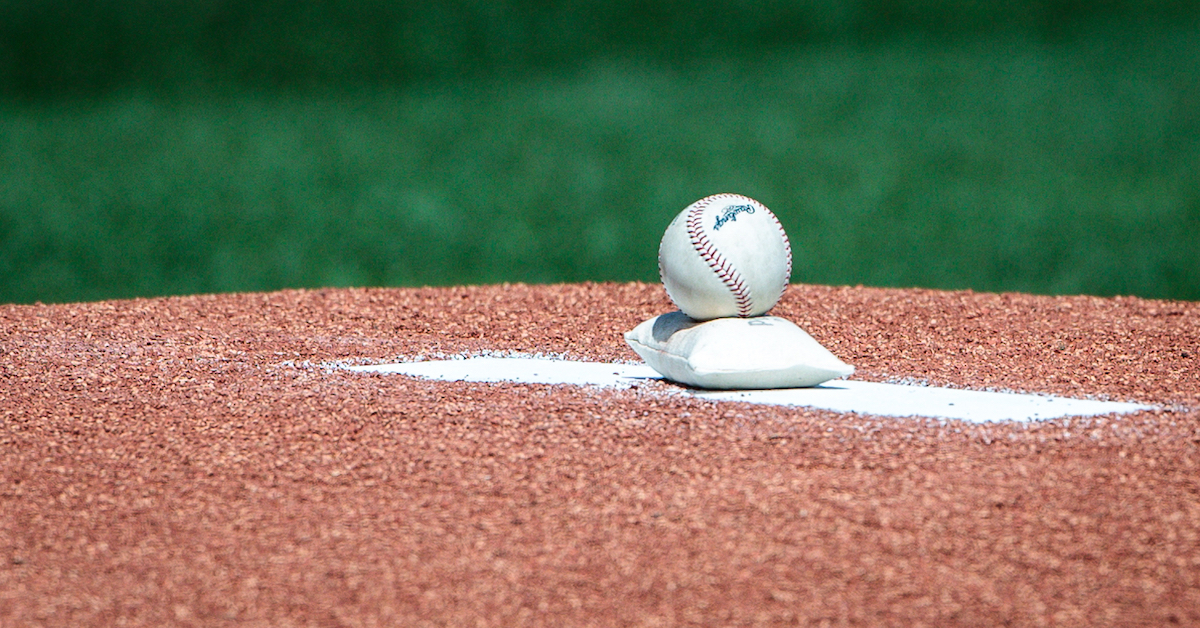
Whether there’s a luddite revolt, a scouting counter-revolution, or another try at rolling again the technological advances the sport has seen in recent times, final week I detailed why it is smart for pitchers to regulate to new expertise proper now. Sure, hurlers may look forward to a tech nullification or, in its absence, a brand new type of tech to degree the taking part in discipline, however as issues stand, the scales are tipping.
Throughout the pitch-tracking period, hurlers have stood to profit greater than hitters from analytics due to extremely customizable pitching plans. In current years, motion-capture programs have helped optimize pitchers’ mechanics along with their repertoires. But this 12 months, a major variety of groups have unearthed one other use of limb-tracking software program: in-game pitch tipping. Essentially, machine studying identifies refined variations in muscle activation in real-time, usually as a result of totally different grips throughout pitch varieties, whereas the pitcher remains to be holding the ball in his glove. After a fast relay system, that info reaches the sphere, and the batter can then look to the dugout or a base coach for some indication of what’s coming.
To counteract this, I recommended pitchers combine up their wrist motion and finger strain proper earlier than launch; by the point the KinaTrax programs decide up what’s happening, it’ll be too late. Sadly, I don’t have entry to metrics like breadth of wrist motion and finger strain relative to grip, so I made a decision to give you my very own approach of figuring out pitchers who’re already doing what I might suggest. I theorized that spin axis could possibly be a proxy for grip, because the level about which a thrown baseball spins is closely reliant on how the pitcher holds it. The path and magnitude of motion can be carefully associated to spin axis, however there isn’t a one-to-one correspondence, so I hypothesized that pitchers with broad variability in motion regardless of minimal spin axis variability could possibly be including some additional mustard after separation.
Unfortunately, the pitchers I recognized — specifically, Martín Pérez and Kevin Gausman — achieved their broad ranges of actions relative to their small ranges of spin axes by way of a mess of various grips (Pérez) or a pair of very totally different grips (Gausman). This was once approach for preserving a batter guessing, given hitters’ reliance on spin axis (or seam orientation) as a cue for pitch motion. The significance of spin axis as a cue is supported by a quantity of research, articles, and hitting clinics, in addition to coaches just like the Yankees’ Dillon Lawson, who in a current interview described how he stresses to his hitters “instead of seeing the ball longer, try to see it earlier” in order that they will decide up on spin and different indicators. But the place Pérez and Gausman could have beforehand discovered success by taking the spin-axis cue away throughout totally different pitch varieties, their variations in grip throughout those self same choices have left them susceptible to motion-capture programs.
So I headed again to the drafting board, in want of a brand new approach to management for grip along with spin. Ultimately, I settled on every pitcher’s motion variability relative to spin axis variability inside single pitch varieties somewhat than throughout total arsenals — the considering behind this being that it’s exceedingly uncommon for a person pitcher to have two totally totally different grips that lead to a pitch of the identical class.
Due to points that come up when working with round statistics (i.e., these given in levels), I separated the angular spin axis into two parts: sine and cosine. In my final piece, I discovered that on the arsenal-level, the usual deviation (SD) of sine was extremely correlated with the SD of horizontal motion, and the SD of cosine with the SD of vertical motion. The similar ought to go for the pitch-level:
And sine:
Something’s not proper right here. Even contemplating I took out the gyro pitches (these with a imply spin effectivity of 25% or much less) that aren’t properly understood by Statcast’s two-dimensional spin axis measure, in addition to pitches with a measurement error (greater than 100% spin effectivity by Alan Nathan’s calculations), the correlations aren’t wherever close to what I used to be getting final week. The main distinction being that I’m categorizing by pitch sort this time.
I’ve lengthy taken concern with MLB’s classification system, which depends on a mixture of supervised and unsupervised machine studying algorithms. By “supervised,” I imply that a few of their algorithmic coaching includes utilizing manually-entered pitch varieties, which the mannequin then bases its future output on. This goes hand-in-hand with MLB’s said need to have pitch varieties on document that match what every pitcher (or their coach) calls the providing, however it’s doubtless what’s messing with the spin axis/motion correlations above — for instance, there’s a lack of standardization when it comes to what a slider means throughout totally different pitchers and coaches.
For the duty at hand, it’s value contemplating MLB’s system as a result of it’s knowledgeable by the pitchers themselves, who’re in flip knowledgeable as to what pitches they’re throwing by their very own grips and wrist motion. Further, within the absence of direct quotes about pitch varieties, MLB makes use of nonetheless photos of pitch grips to tell their classifications. Still, I selected to match their classifications to my very own mannequin to function a verify each on MLB’s system and the concept that there’s nonetheless an important relationship between spin axis and motion on the pitch-level. This will in flip inform how I’m going about investigating which pitchers are greatest geared up to outlive the brand new wave of pitch tipping expertise.
There have been myriad makes an attempt at unsupervised classification, however I drew most from Professor Brian Mills’ work. His demonstration of model-based clustering appealed to me probably the most as a result of his mannequin chooses its personal variety of groupings for every pitcher (although I capped the potential variety of pitches at 9 for computational functions and since these are the max that MLB has anybody — Yu Darvish — throwing), its personal minimization criterion (from 14 totally different choices) for every pitcher primarily based on the form of their pitch distribution throughout the related variables (standardizing for density as an alternative), and the place within the pitch-cluster house to begin optimizing from. The solely different limitation I imposed was a Bayesian prior in an effort to normalize the distribution of the variety of pitch varieties per pitcher.
For MLB’s mannequin, as soon as every pitcher has a big sufficient massive league pattern, they’re categorized primarily based on a personalized neural community skilled solely on their pitches, which may make the groupings legitimate at the very least for comparisons inside every pitcher. This is the same concept to permitting for a (customizable) number of totally different minimization standards and beginning factors within the optimization drawback; the foremost philosophical distinction between the 2 fashions is that mine is selecting its personal variety of pitch varieties for every pitcher. It does so with none enter from the pitcher, solely primarily based on the distributions of velocity, spin price, spin axis in three dimensions (sine, cosine, and spin effectivity), and horizontal and vertical motion.
The draw back to this technique is that there is no such thing as a direct grip-based enter, however it makes up for that in not requiring every pitcher to have a excessive degree of self-awareness; a slight tweak they make for a slower or tighter model of a breaking ball, for instance, would possibly trigger the pitch to diverge into two varieties in my mannequin whereas MLB nonetheless considers it beneath one umbrella. On the opposite hand, there could also be some cases by which a pitcher thinks they throw two totally different breaking balls, however my mannequin disagrees.
It seems that the previous was extra widespread than the latter. Among the 248 pitchers who threw at the very least 500 pitches by way of Saturday, my mannequin recognized 831 distinct pitch varieties thrown at the very least 100 instances, whereas MLB has recognized 773, with each figures together with gyro pitches, of which there have been 186 and 163, respectively. The added flexibility in my mannequin resulted in higher correlations between cosine and vertical motion (r-squared of .49 vs. .24):
And sine and horizontal motion (.28 and .07):
As properly as, for gyro pitches, spin effectivity and vertical motion (.50 and .14):
And spin effectivity and horizontal motion had been nearly the identical (.58 and .56):
Note that the scales are totally different on all of those graphs — it’s extra aesthetically pleasing that approach, however it’s additionally the explanation among the variations in correlations look smaller or bigger than they really are. Another transfer I made for aesthetically-pleasing functions was eradicating the one main outlier — a Rich Hill (nobody is shocked) cluster that my mannequin recognized. Removing it really worsened the correlations for my mannequin, however that cluster was actually on the market: its horizontal motion and vertical motion SDs had been each almost 4 inches higher than the second-highest. It additionally had the very best normal deviation of spin axis sine and cosine, in addition to spin effectivity.
This is an instance of my mannequin battling the truth that Hill has thrown eight changeups, 36 sinkers, and three sliders this season. All three of these pitches have at the very least one thing in widespread with a subset of his cutters (of which he’s thrown 208): the sinker and changeup have comparable velocities (low 80s) and comparable quantities of rise (from six to eight inches), whereas the slider has the same spin effectivity (32.9% vs. the cutter’s 17.2%) and spin price (2421 vs. 2305 rpms). It so occurred that optimization on this case meant lumping all of these choices collectively; usually, curbing the variety of pitch varieties produced higher options for my mannequin, however not on this case.
Despite the occasional wayward resolution granted by way of unsupervised studying, I nonetheless assume that my mannequin (or the same one) ought to be utilized in live performance with MLB’s pitch classification system as a result of the latter merely doesn’t do one of the best job at grouping pitches based on the traits that underlie pitch motion and batter pitch recognition. Yet, to in the end determine the pitchers who can climate advances in pitch tipping, we have to take a look at motion variability within the context of spin axis (i.e., which pitchers can take away the spin cue for hitters) and grip; the worth in MLB’s system is that it serves as one of many solely methods to issue grip into the equation. Stay tuned for extra on the pitchers who put collectively one of the best of each worlds.
Content Source: blogs.fangraphs.com