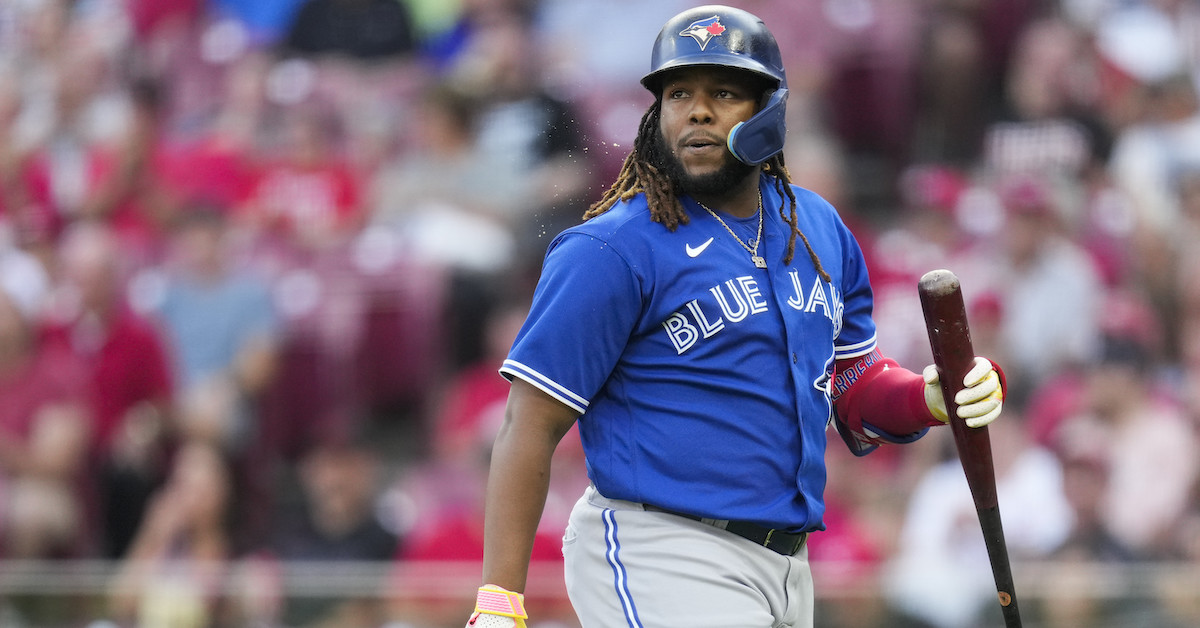
Some of probably the most irritating arguments involving baseball statistics revolve round the usage of anticipated stats. Perhaps probably the most continuously cited of those metrics are Statcast’s xStats, which use Statcast information for hitters to estimate the batting common, on-base share, slugging share, and wOBA you’d “expect” a hitter to realize. Investigating how predictive xStats are in comparison with their corresponding precise stats has been a standard analysis train over the previous couple of years. While it will depend on the precise dataset used, xStats by themselves typically aren’t significantly better than the precise stats at predicting the following 12 months’s precise stats. But that doesn’t imply we should always merely discard anticipated stats when making an attempt to judge gamers.
While I’m not going spend an excessive amount of time speaking about how predictive xStats are versus the precise ones, I do wish to briefly contact on a number of the present work on the topic. Jonathan Judge at Baseball Prospectus examined lots of the anticipated metrics again in 2018. He additionally spoke with MLBAM’s Tom Tango concerning the nature of anticipated stats and their utilization:
Earlier this week, we reached out to BAM with our findings, asking if they’d any remark.
MLBAM Senior Database Architect of Stats Tom Tango promptly responded, asking that we guarantee we had the latest model of the information, as a result of some current modifications being made. We refreshed our information units, discovered some small modifications, and retested. The outcomes had been the identical.
Tango then burdened that the anticipated metrics had been solely ever meant to be descriptive, that they weren’t designed to be predictive, and that if they’d been meant to be predictive, they may have been designed otherwise or different metrics may very well be used.
One of my colleagues, Jeff Zimmerman, wrote about xStats within the fantasy context in 2018. Justin Mason appeared into the information in 2021 and located that xStats are much less predictive than precise ones.
It’s all the time good to have probably the most up-to-date data, so let’s begin there. I pulled each participant with consecutive 200 PA seasons since 2015; there have been simply over 1,800 season-pairs. I then ran the r-squared (the coefficient of dedication) for xBA, xOBP, xSLG, xwOBA and their noticed counterparts within the first season, and in contrast it to the second season:
R-Squared, xStats vs. Actual
Relationship | R-Squared |
---|---|
xBA to Next Year BA | 0.173 |
BA to Next Year BA | 0.163 |
xOBP to Next Year OBP | 0.236 |
OBP to Next Year OBP | 0.210 |
xSLG to Next Year SLG | 0.226 |
SLG to Next Year SLG | 0.189 |
xwOBA to Next Year wOBA | 0.221 |
wOBA to Next Year wOBA | 0.179 |
One factor that’s value noting with this information set, which comes proper as much as Monday morning, is that I’m getting barely higher correlations than others have gotten up to now. The reason behind that’s tough to determine, although one potential clarification is that the change from Trackman to Hawk-Eye in 2020 has helped to enhance these metrics.
Still, the connection between the anticipated stats and the precise ones is barely barely stronger than the choice. That doesn’t imply, nonetheless, that the anticipated stats don’t matter when making evaluations.
When developing a mannequin, a developer will interact in a course of referred to as “dimensionality reduction.” There are many strategies for doing this, however the fundamental thought is to take a dataset and scale back the variety of options whereas nonetheless preserving the validity of the mannequin. One factor that every one the strategies share, nonetheless, is that they don’t merely throw out variables as a result of they’ve comparable and even lesser correlations with the dependent variable. Even a variable that performs worse than one other can nonetheless contribute to creating a mannequin extra correct than it could be in any other case. This will not be an unusual prevalence.
Imagine you’re making an attempt to mannequin somebody’s life expectancy. Age is a particularly essential variable. But components akin to whether or not the individual is a smoker, their socioeconomic standing, and their well being historical past are additionally variables that, if recognized, serve to make the mannequin extra correct than merely utilizing age alone. The secret’s figuring out whether or not these lesser variables are capturing some helpful data that age alone will not be. Let’s use a baseball instance to display this.
Since the divisional period began in 1969, the r-squared for OBP vs. runs per sport is 0.73, mainly that means that 73% of the noticed variance in OBP explains the noticed variance in runs per sport. For SLG, that quantity is 0.82. Team OBP has a weaker relationship with runs per sport than Team SLG, however utilizing each makes the mannequin much better. OPS’ r-squared is 0.905, and OBP*SLG is 0.911. Now take the final a part of the triple-slash, batting common. The r-squared for BA and runs per sport is 0.53, however on this case, it’s not including data that OBP and SLG aren’t already capturing; the r-squared for a mannequin of runs scored per sport solely improves to 0.914 when incorporating BA. Indeed, when OBP and SLG are used, BA is definitely a really slight destructive issue, as a result of OBP/SLG mixtures barely underrate walks.
So the pertinent query isn’t whether or not xStats are higher than precise stats at predicting future efficiency, however whether or not they enhance our capability to foretell future efficiency when used at the side of precise stats. Below are the RMSE (root-mean squared error) for the related stats:
RMSE for Expected Stats vs. Actual
Stat | RMSE |
---|---|
xBA to Next Year BA | 0.0347 |
BA to Next Year BA | 0.0317 |
xBA and BA to Next Year BA | 0.0312 |
xOBP to Next Year OBP | 0.0370 |
OBP to Next Year OBP | 0.0350 |
xOBP and OBP to Next Year OBP | 0.0345 |
xSLG to Next Year SLG | 0.0760 |
SLG to Next Year SLG | 0.0739 |
xSLG and SLG to Next Year SLG | 0.0719 |
xwOBA to Next Year wOBA | 0.0403 |
wOBA to Next Year wOBA | 0.0385 |
xWOBA and wOBA to Next Year wOBA | 0.0378 |
Knowing the error ranges of those stats doesn’t immediately inform a consumer how you can deal with this information. So somewhat than ask what the error of every stat is, I went again to the complete dataset and as an alternative requested what linear mixture of the xStat and the precise stat have labored greatest:
Mixing xStats and Actual Stats
Stat | xStat | Actual Stat |
---|---|---|
Next Year BA | 73% | 27% |
Next Year OBP | 70% | 30% |
Next Year SLG | 59% | 41% |
Next Year wOBA | 65% | 35% |
As a easy rule of thumb, you gained’t do too badly if you happen to merely regress xStats a 3rd of the best way in the direction of the precise ones. But as you might need guessed, that modifications relying on the participant’s variety of plate appearances.
For BA, if you happen to solely take a look at the gamers with no less than 600 plate appearances within the first season, the best BA combine is 37% BA, 63% xBA. When you solely take a look at the gamers with between 200 and 300 plate appearances, that turns into 10% BA and 90% xBA, a drastically totally different quantity. Naturally, this displays the truth that the longer a participant outperforms their xStats, the nearer to the precise stats you count on them to be sooner or later. But let’s calculate that, too.
Adding a number of 12 months inputs into the combination, I calculated the stabilization level for every of those 4 stats. This is the variety of plate appearances at which the xStat and the precise stat have equal predictive energy:
Stabilization Points for Expected Stats vs. Actual Stats
Stat | Stabilization Point (PA) |
---|---|
BA | 1154 |
OBP | 1007 |
SLG | 607 |
wOBA | 766 |
Sticking with utilizing solely xStats and precise ones to foretell the longer term, you may approximate how a lot of the particular stat to make use of with the formulation Actual % = PA / (PA + Stablization Point).
If you’re making an attempt to renovate your own home, you may’t use a screwdriver for each job. But if you happen to throw away your screwdriver as a result of there’s so much it may possibly’t do, you’ll remorse it once you encounter a screw. xStats aren’t a predictive mannequin by themselves, however they could be a essential a part of a predictive mannequin. The zStats utilized in ZiPS take a look at issues like spray tendencies and pace to enhance accuracy, however xStats are nonetheless a useful gizmo.
I’ll take a look at the pitcher facet of the equation in a future piece.
Content Source: blogs.fangraphs.com