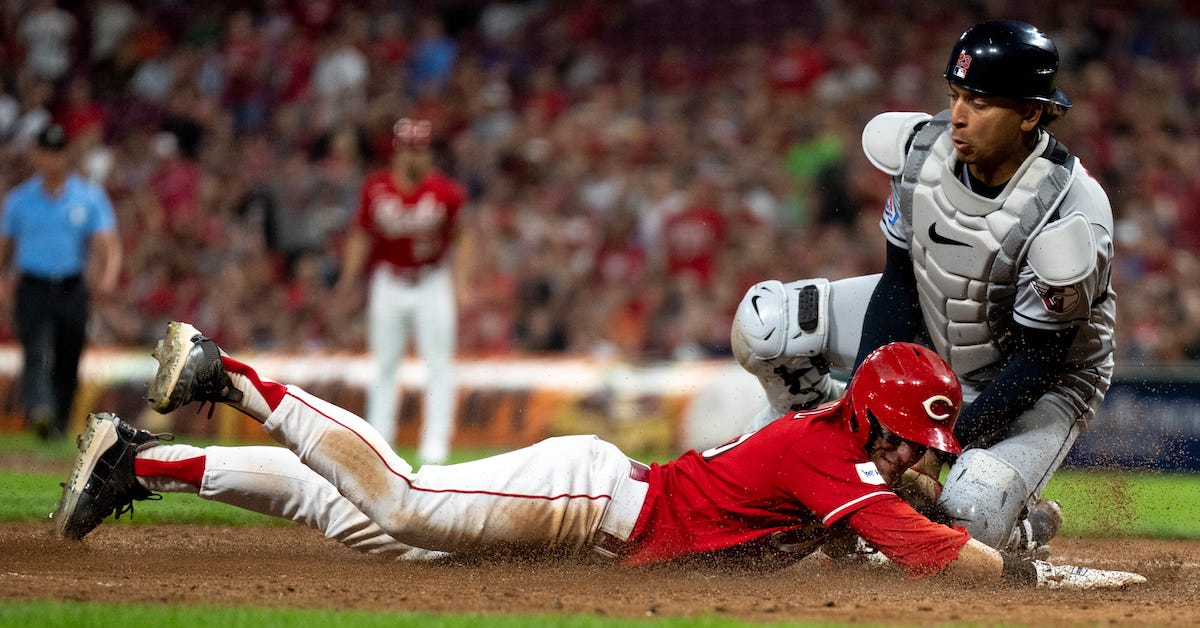
Look, I do know that isn’t the road. It’s a hen in each pot. But I got here up with a superb lobster pun final week, and I’m writing extra in regards to the ins and outs of groups driving house runners from third base, so I made a decision to return to the properly. You’ll simply must stay with it; I’m the one driving the boat right here, and because it seems, it’s a lobster boat.
With the puns are actually settled, let’s get all the way down to enterprise. Last week, I chopped up the 2023 season into halves to see how properly varied statistical indicators correlated with a workforce’s future skill to money of their runners. As a recap, strikeout fee had a reasonably robust correlation, and never a lot else did. Quite frankly, although, I wasn’t significantly satisfied by that. There simply wasn’t sufficient knowledge. With solely 30 observations, it’s too simple for one workforce to skew issues, or not less than that’s the way it feels in my head.
There’s a straightforward resolution: extra knowledge. So I used the identical split-half methodology from final week and began chopping previous seasons in two. More particularly, I picked the years from 2012-22, excluding the shortened 2020 season. In every case, I adopted the identical process: I break up the season in two and famous every workforce’s offensive statistics within the first half. Then I checked out how environment friendly every workforce was at scoring when a runner reached third with lower than two outs. I received a a lot larger pattern this time; 300 observations, which makes it so much more durable for a single outlier to mess issues up.
As it seems, a single outlier may need been messing issues up. Over this a lot bigger pattern, each main statistical measure of offensive efficiency did fairly “poorly,” not less than inasmuch as they predicted little or no of a workforce’s subsequent skill to transform runners on third with lower than two outs into runs. In an effort to preserve phrases and never must sort that lengthy and complicated phrase each time I need to speak about this idea, let’s simply name that “conversion rate.”
So yeah… conversion fee is a difficult factor to foretell. Here, to your tabular enjoyment, are the correlations between varied first-half statistics and second-half conversion fee, in addition to r-squared’s in case you don’t really feel like doing the maths your self:
Correlations with Conversion Rate for Various Stats
Statistic | Correlation | R-Squared |
---|---|---|
BB% | 0.087 | 0.008 |
Okay% | -0.121 | 0.014 |
ISO | 0.101 | 0.01 |
BABIP | 0.004 | 0 |
AVG | 0.102 | 0.01 |
OBP | 0.136 | 0.018 |
SLG | 0.126 | 0.016 |
wOBA | 0.137 | 0.019 |
wRC+ | 0.124 | 0.015 |
In plain English: blah. None of that is all that fascinating. Want to show your good offensive conditions into runs extra ceaselessly? Just hit for common… or energy… or get on base so much… or don’t strike out… or simply have a usually good offense. The highest correlation between first-half efficiency and second-half conversion fee is wOBA, which simply occurs to be probably the most fundamental “is your team good at hitting” quantity out there. It does only a hair higher than wRC+, which is smart to me; wRC+ adjusts for park results, however “did you score a run” and wOBA each don’t. In my future evaluation, I made a decision to throw out BABIP and wRC+; they didn’t appear to be bringing a lot to the social gathering.
So must you simply quit, go the nihilistic route, and say that nothing may help you perceive which groups will submit the most effective conversion charges? You may, if you would like, in the best way that the reply to a ton of baseball questions appears to be “random variance.” But that’s not a very satisfying reply, and there are actual results right here; higher offensive groups actually do convert at a better fee. So I attempted just a few different strategies to attempt to get on the coronary heart of the issue.
First, I ran two-variable correlations for each mixture of two core offensive statistics. I didn’t have a ton of hope that this is able to give me fascinating knowledge, however it’s value attempting one thing even if you happen to aren’t positive it’ll work simply to rule it out. Bad information, although: it didn’t work. I hope you want lengthy, mostly-meaningless tables with far too many knowledge factors:
Correlations with Conversion Rate for Various Stat Pairs
Stat 1 | Stat 2 | Correlation 1 | Correlation 2 | R^2 |
---|---|---|---|---|
BB% | Okay% | 0.522 | -0.289 | 0.028 |
BB% | ISO | 0.236 | 0.164 | 0.012 |
BB% | AVG | 0.428 | 0.378 | 0.02 |
BB% | OBP | 0.106 | 0.414 | 0.019 |
BB% | SLG | 0.244 | 0.178 | 0.019 |
BB% | wOBA | 0.17 | 0.377 | 0.02 |
Okay% | ISO | -0.305 | 0.293 | 0.033 |
Okay% | AVG | -0.184 | 0.161 | 0.016 |
Okay% | OBP | -0.164 | 0.355 | 0.024 |
Okay% | SLG | -0.218 | 0.188 | 0.028 |
Okay% | wOBA | -0.178 | 0.349 | 0.026 |
ISO | AVG | 0.17 | 0.279 | 0.016 |
ISO | OBP | 0.097 | 0.381 | 0.02 |
ISO | SLG | -0.11 | 0.279 | 0.016 |
ISO | wOBA | 0.011 | 0.413 | 0.019 |
AVG | OBP | -0.059 | 0.501 | 0.019 |
AVG | SLG | 0.11 | 0.17 | 0.016 |
AVG | wOBA | -0.048 | 0.459 | 0.019 |
OBP | SLG | 0.317 | 0.089 | 0.02 |
OBP | wOBA | 0.209 | 0.249 | 0.019 |
SLG | wOBA | -0.007 | 0.437 | 0.019 |
These are higher, however not by a lot. And the pairs that specify issues probably the most aren’t those I anticipated. Walk fee and strikeout fee? Strikeout fee and ISO? It makes excellent sense to me that strikeout fee is a key a part of the puzzle, however what goes with it doesn’t actually monitor. One small word: due to the best way I pulled the info by aggregating outcomes, I couldn’t embrace fly ball fee, however I’m guessing that ISO is one thing of a proxy for it, maybe with different data on hard-hit fee as properly.
I didn’t need to cease there, however we’re getting outdoors of the realm of simply interpretable options. A couple of commenters final week advised options: dominance evaluation, relative weights evaluation, principal elements evaluation, and perhaps some others that I missed. I used to be already accustomed to PCA weighting from previous expertise, however it didn’t actually match with what I used to be in search of. The different two appeared considerably promising, but in addition phenomenally exhausting to interpret. Then I had an epiphany: If my output goes to be exhausting to interpret anyway, why not simply chuck it right into a machine studying algorithm and see what comes out?
Bad information on that entrance: I chucked it into a wide range of machine studying algorithms, and never a lot got here out. Chewing up all that knowledge without delay in a multivariate regression did about in addition to I did by myself, with a negligible r-squared. Only strikeout fee gave the impression to be important, and never very important at that. I began throwing every thing I had at it, together with attempting to interpret these strategies from up above. Relative weights evaluation gave ISO, common, and slugging share excessive weights (with slugging having inverse correlation), which seems like gibberish to me. PCA remodeled the info into three uninterpretable principal elements, and nonetheless solely produced an r-squared about nearly as good as utilizing strikeout fee and ISO.
I hate to say it, however I don’t assume I can discover any clear patterns regardless of all of this additional knowledge. As an added flourish, the year-to-year correlation between groups’ skill to drive runners house from third with lower than two outs is actually zero, so it’s not like there’s even a lot proof that groups have cracked the code. I’m nonetheless hopeful that one thing will shake out, however I’ve no clue the place. Hey, perhaps you possibly can assist! Here’s a spreadsheet with the info I smashed into varied fashions within the earlier part. If you are feeling like messing round with it, go nuts.
In the tip, maybe the most important message right here is the shortage of predictability. You can’t tease out what’s going to occur on the sphere primarily based on broad aggregates. You can’t forecast what’s going to occur within the greatest moments now primarily based on what occurred within the greatest moments prior to now. The sport as we speak is what issues. No one is doomed to strand these runners, or fated to money them in. The method you win is by executing every day, not by cashing in on some innate trait that makes you extra more likely to do the clutch factor. I discover that comforting. Even on this age of huge knowledge and omnipresent cameras, gamers doing their factor higher remains to be a very powerful issue, and who these guys are can and does change from someday to the subsequent.
Content Source: blogs.fangraphs.com