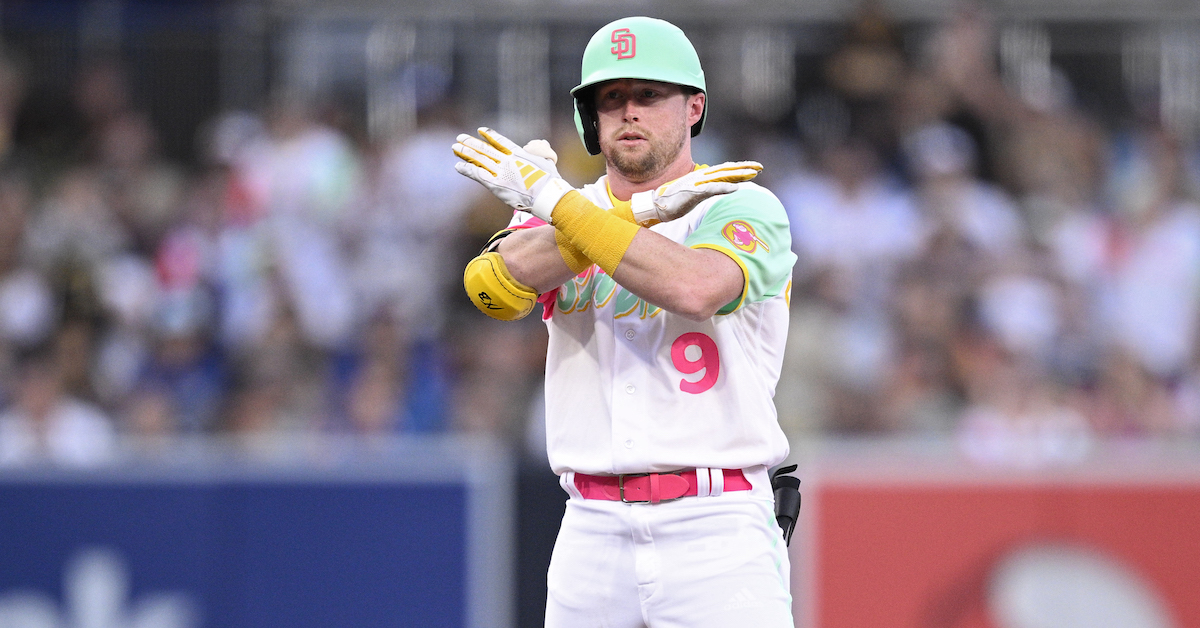
One of the unusual issues about projecting baseball gamers is that even outcomes themselves are small samples. Full seasons lead to particular numbers which have minimal predictive worth, reminiscent of BABIP for pitchers. The predictive worth isn’t actually zero — particular person seasons kind a lot of the idea of projections, whether or not math-y ones like ZiPS or just our private opinions on how good a participant is — however we now have to develop instruments that enhance our skill to clarify a few of these stats. It’s not sufficient to know that the variety of residence runs allowed by a pitcher is unstable; we have to know the way and why pitchers permit homers past a common sense of pitching poorly or being Jordan Lyles.
Data like that which StatCast supplies offers us the flexibility to get at what’s extra elemental, reminiscent of exit velocities and launch angles and the like — issues which are in themselves extra predictive than their finish merchandise (the variety of homers). StatCast has its personal implementation of this sort of train in its varied “x” stats. ZiPS makes use of barely totally different fashions with an analogous function, which I’ve dubbed zStats. (I’m going to make you guess what the z stands for!) The variations within the fashions will be important. For instance, when speaking about grounders, balls hit straight towards the second base bag turned singles 48.7% of the time from 2012 to ’19, with 51.0% outs and 0.2% doubles. But grounders hit 16 levels to the “left” of the bag solely turned hits 10.6% of the time over the identical stretch, and towards the second base aspect, it was 9.8%. ZiPS makes use of knowledge like dash pace when calculating hitter BABIP, as a result of how briskly a participant is has an impact on BABIP and extra-base hits.
ZiPS doesn’t discard precise stats; the fashions all enhance from understanding the precise numbers along with the zStats. You can learn extra on how zStats relate to precise stats right here. For these curious in regards to the r-squared values between zStats and actual stats for the offensive parts, it’s 0.59 for zBABIP, 0.86 for strikeouts, 0.83 for walks, and 0.78 for homers. Those relationships are what make these stats helpful for predicting the long run. If you’ll be able to clarify 78% of the variance in residence run price between hitters with no details about what number of homers they really hit, you’ve answered a variety of the riddle. All of those numbers correlate higher than the precise numbers with future numbers, although a mannequin that makes use of each zStats and precise ones, as the total mannequin of ZiPS does, is superior to both by themselves.
And why is that this necessary and never simply number-spinning? Knowing that modifications in stroll charges, residence run charges, and strikeout charges stabilized far faster than different stats was an necessary step ahead in participant valuation. That’s one thing that’s helpful whether or not you’re employed for a entrance workplace, are a hardcore fan, wish to make some fantasy league strikes, and even only a common fan who’s rooting in your faves. If we enhance our information of the fundamental molecular construction of a stroll or a strikeout, then we will discover gamers who’re enhancing or struggling much more shortly, and supply higher solutions on why a stroll price or a strikeout price has modified. This is helpful knowledge for me particularly as a result of I clearly do a variety of work with projections, however I’m hoping the sort of data is attention-grabbing to readers past that.
As with any mannequin, the proof of the pudding is within the consuming, and there are all the time some folks that query the worth of knowledge reminiscent of these. So for this run, I’m pitting zStats in opposition to the final two months and all new knowledge that clearly couldn’t have been used within the mannequin and not using a time machine to see how the zStats did in comparison with actuality. I’m not going to do a complete submit for this each time, however that is one thing that, based mostly on the suggestions from the final submit in June, individuals actually needed to see the outcomes for.
Starting with zBABIP, let’s take a look at how the numbers have shaken out for the leaders and trailers from again in June. I didn’t embrace gamers with fewer than 100 plate appearances over the past two months.
zBABIP Overachievers (As of 6/8)
zBABIP Underachievers (As of 6/8)
For the overachievers, when it got here to predicting BABIP for the middle-third of the season, zBABIP was nearer than precise BABIP for 19 of the 23 gamers projected, with a imply absolute error (MAE) of 42 factors of BABIP versus 72 factors. For the underachivers, zBABIP was nearer on 16 of 20 with an MAE of 43 factors versus 65 factors.
On to homers.
zHR Overachievers (As of 6/8)
zHR Underachievers (As of 6/8)
Closer victories for ZiPS right here, which isn’t shocking as a result of homers is solely a extra predictive stat than zBABIP! zHR price was nearer than precise on 12 of the 23 overachievers and 15 of 23 underachievers. The MAEs have been additionally two nearer wins, with 2.1% versus 2.6% on the primary group and 1.3% versus 1.7% on the second. Ohtani nearly singlehandedly received it for the primary group along with his homer surge!
zBB Overachievers (As of 6/8)
Name | BB | zBB | zBB Diff | BB% (6/8) | zBB% (6/8) | BB% Since |
---|---|---|---|---|---|---|
Jake Cronenworth | 32 | 19.6 | 12.4 | 12.5% | 7.6% | 5.5% |
Ryan Noda | 42 | 31.5 | 10.5 | 19.8% | 14.9% | 14.0% |
Ian Happ | 45 | 35.0 | 10.0 | 17.0% | 13.2% | 15.4% |
Byron Buxton | 26 | 17.5 | 8.5 | 12.3% | 8.3% | 6.7% |
Joey Gallo | 25 | 17.2 | 7.8 | 15.2% | 10.4% | 10.4% |
Juan Soto | 56 | 48.4 | 7.6 | 20.9% | 18.1% | 19.2% |
Myles Straw | 21 | 14.0 | 7.0 | 9.4% | 6.3% | 6.9% |
Hunter Renfroe | 17 | 10.1 | 6.9 | 6.9% | 4.1% | 8.0% |
Willson Contreras | 24 | 17.1 | 6.9 | 10.1% | 7.2% | 9.4% |
Nico Hoerner | 17 | 10.3 | 6.7 | 6.9% | 4.2% | 5.6% |
Matt Olson | 44 | 37.6 | 6.4 | 15.7% | 13.4% | 11.3% |
José Abreu | 17 | 10.6 | 6.4 | 6.7% | 4.2% | 6.8% |
Kyle Schwarber | 44 | 37.7 | 6.3 | 16.6% | 14.2% | 15.4% |
Andrew McCutchen | 35 | 29.1 | 5.9 | 15.5% | 12.9% | 17.1% |
Adley Rutschman | 45 | 39.3 | 5.7 | 16.5% | 14.4% | 9.6% |
Josh Bell | 30 | 24.3 | 5.7 | 13.0% | 10.5% | 9.0% |
Alejandro Kirk | 20 | 14.7 | 5.3 | 11.3% | 8.3% | 7.0% |
Will Smith | 28 | 22.8 | 5.2 | 14.8% | 12.1% | 12.6% |
Miguel Cabrera | 12 | 6.8 | 5.2 | 10.7% | 6.1% | 10.0% |
MJ Melendez | 26 | 21.0 | 5.0 | 11.1% | 8.9% | 7.7% |
Jean Segura | 15 | 10.1 | 4.9 | 7.4% | 5.0% | 5.6% |
zBB Underachievers (As of 6/8)
Name | BB | zBB | zBB Diff | BB% (6/8) | zBB% (6/8) | BB% Since |
---|---|---|---|---|---|---|
Esteury Ruiz | 10 | 20.0 | -10.0 | 3.7% | 7.3% | 3.8% |
Luis García | 13 | 21.5 | -8.5 | 5.6% | 9.3% | 4.5% |
Mike Yastrzemski | 13 | 20.8 | -7.8 | 7.6% | 12.2% | 13.8% |
Connor Joe | 21 | 28.7 | -7.7 | 10.7% | 14.6% | 7.8% |
Francisco Alvarez | 7 | 14.5 | -7.5 | 5.1% | 10.6% | 8.3% |
Corey Julks | 4 | 10.6 | -6.6 | 2.4% | 6.3% | 12.4% |
Bo Bichette | 13 | 19.5 | -6.5 | 4.5% | 6.8% | 3.7% |
J.D. Martinez | 10 | 16.5 | -6.5 | 4.9% | 8.1% | 9.6% |
Bryan Reynolds | 21 | 27.5 | -6.5 | 8.3% | 10.9% | 7.3% |
Ozzie Albies | 14 | 20.1 | -6.1 | 5.4% | 7.8% | 8.7% |
TJ Friedl | 10 | 16.1 | -6.1 | 6.3% | 10.2% | 8.7% |
Rafael Devers | 16 | 21.6 | -5.6 | 6.2% | 8.4% | 10.8% |
Michael A. Taylor | 9 | 14.6 | -5.6 | 5.3% | 8.6% | 4.8% |
Emmanuel Rivera | 5 | 10.1 | -5.1 | 5.1% | 10.2% | 7.4% |
Jorge Soler | 24 | 28.9 | -4.9 | 9.8% | 11.7% | 12.7% |
Leody Taveras | 13 | 17.9 | -4.9 | 7.1% | 9.8% | 4.6% |
Taylor Ward | 18 | 22.9 | -4.9 | 7.3% | 9.3% | 12.5% |
Bobby Witt Jr. | 11 | 15.6 | -4.6 | 4.2% | 5.9% | 6.1% |
Ronald Acuña Jr. | 29 | 33.3 | -4.3 | 10.2% | 11.8% | 12.9% |
Unusually, ZiPS did considerably higher with pegging overachievers than underachievers. You don’t see it within the fundamental win totals (13 of 21 and 11 of 19), however zBB had a major benefit in MAE for the overachievers (2.0% versus 3.0%) and was very shut in underachievers (2.9% versus 3.1%). zBB shouldn’t be kicking butt right here; the worth of zBB is along with precise stroll price, not as a substitute of. zBB and precise BB are a giant acquire when utilized in tandem.
zSO Overachievers (As of 6/8)
Name | SO | zSO | zSO Diff | SO% (6/8) | zSO% (6/8) | SO% Since |
---|---|---|---|---|---|---|
Masataka Yoshida | 24 | 38.6 | -14.6 | 9.9% | 15.9% | 13.8% |
Brent Rooker | 60 | 74.1 | -14.1 | 26.9% | 33.2% | 39.2% |
Esteury Ruiz | 47 | 61.0 | -14.0 | 17.2% | 22.3% | 21.9% |
Andrew McCutchen | 44 | 57.3 | -13.3 | 19.5% | 25.4% | 22.4% |
Max Muncy | 68 | 81.1 | -13.1 | 28.6% | 34.1% | 24.7% |
Paul Goldschmidt | 57 | 69.9 | -12.9 | 20.9% | 25.6% | 22.1% |
Randy Arozarena | 60 | 72.8 | -12.8 | 22.9% | 27.8% | 25.9% |
Harold Ramírez | 36 | 48.5 | -12.5 | 19.3% | 25.9% | 17.7% |
Pete Alonso | 51 | 63.3 | -12.3 | 19.5% | 24.3% | 22.3% |
Javier Baez | 49 | 60.6 | -11.6 | 20.5% | 25.4% | 23.2% |
Gleyber Torres | 33 | 44.1 | -11.1 | 12.3% | 16.5% | 14.4% |
Bryan Reynolds | 47 | 58.0 | -11.0 | 18.7% | 23.0% | 23.0% |
Andrew Vaughn | 47 | 57.7 | -10.7 | 18.1% | 22.3% | 23.6% |
Jose Siri | 43 | 53.4 | -10.4 | 32.8% | 40.8% | 39.4% |
Harrison Bader | 13 | 23.1 | -10.1 | 13.7% | 24.3% | 17.1% |
C.J. Cron | 35 | 45.0 | -10.0 | 23.6% | 30.4% | 20.2% |
Christian Walker | 47 | 56.9 | -9.9 | 19.3% | 23.3% | 18.8% |
Adam Frazier | 23 | 32.4 | -9.4 | 10.4% | 14.7% | 15.0% |
Starling Marte | 40 | 49.4 | -9.4 | 17.8% | 22.0% | 24.0% |
Nick Castellanos | 63 | 72.3 | -9.3 | 24.0% | 27.6% | 29.8% |
José Ramírez | 22 | 31.2 | -9.2 | 8.4% | 12.0% | 11.7% |
Andrés Giménez | 37 | 46.2 | -9.2 | 15.9% | 19.9% | 20.8% |
Will Smith | 19 | 28.2 | -9.2 | 10.1% | 14.9% | 21.3% |
William Contreras | 41 | 50.1 | -9.1 | 20.4% | 24.9% | 17.5% |
zSO Underachievers (As of 6/8)
Name | SO | zSO | zSO Diff | SO% (6/8) | zSO% (6/8) | SO% Since |
---|---|---|---|---|---|---|
Jake Cronenworth | 60 | 41.1 | 18.9 | 23.3% | 16.0% | 13.6% |
Brandon Marsh | 64 | 49.4 | 14.6 | 31.1% | 24.0% | 28.3% |
Thairo Estrada | 50 | 35.6 | 14.4 | 22.5% | 16.0% | 27.1% |
Lane Thomas | 64 | 51.4 | 12.6 | 25.0% | 20.1% | 27.5% |
Taylor Ward | 54 | 41.6 | 12.4 | 22.0% | 16.9% | 15.5% |
Jarred Kelenic | 76 | 63.6 | 12.4 | 32.6% | 27.3% | 32.6% |
Jarren Duran | 52 | 40.2 | 11.8 | 29.1% | 22.5% | 19.5% |
DJ LeMahieu | 59 | 47.4 | 11.6 | 26.7% | 21.4% | 16.8% |
Brandon Belt | 63 | 51.5 | 11.5 | 36.4% | 29.8% | 31.2% |
Nathaniel Lowe | 57 | 46.0 | 11.0 | 20.7% | 16.7% | 23.2% |
Myles Straw | 44 | 33.6 | 10.4 | 19.7% | 15.1% | 20.1% |
Josh Jung | 67 | 57.2 | 9.8 | 27.0% | 23.1% | 31.9% |
Anthony Volpe | 74 | 64.3 | 9.7 | 30.6% | 26.6% | 23.9% |
Brice Turang | 48 | 38.4 | 9.6 | 27.1% | 21.7% | 13.9% |
Seiya Suzuki | 53 | 44.2 | 8.8 | 26.1% | 21.8% | 23.8% |
Ha-Seong Kim 김하성 | 53 | 44.5 | 8.5 | 24.7% | 20.7% | 14.6% |
Byron Buxton | 61 | 52.6 | 8.4 | 28.8% | 24.8% | 35.6% |
More minor wins for zSO each within the easy head-to-head matchups (15 of 24 and 9 of 17) and in MAE (3.5% versus 4.3% and 5.3% versus 5.8%). I used to be particularly concerned with Jake Cronenworth’s outcomes as a result of he had a bizarre outlier bunch of leads to these numbers as each the most important stroll overachiever and the most important strikeout underachiever. And each of those numbers did rebound significantly into issues that ZiPS anticipated. If not, I’d actually should dig farther into his plate self-discipline method to see why his outcomes have been so atypical given his plate self-discipline numbers.
Tomorrow, I’ll have the up to date hitter numbers by August 8.
Content Source: blogs.fangraphs.com