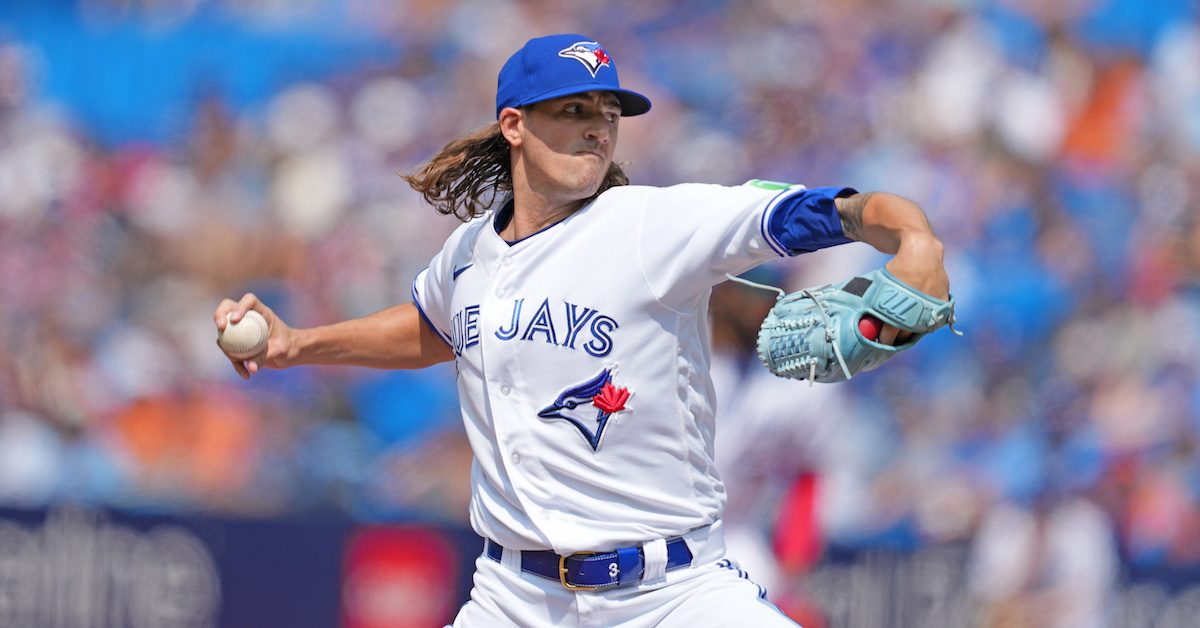
One of the unusual issues about projecting baseball gamers is that even outcomes themselves are small samples. Full seasons end in particular numbers which have minimal predictive worth, resembling BABIP for pitchers. The predictive worth isn’t actually zero — particular person seasons kind a lot of the premise of projections, whether or not math-y ones like ZiPS or just our private opinions on how good a participant is — however we now have to develop instruments that enhance our capability to clarify a few of these stats. It’s not sufficient to know that the variety of dwelling runs allowed by a pitcher is risky; we have to know the way and why pitchers permit homers past a common sense of pitching poorly or being Jordan Lyles.
Data like that which StatCast supplies provides us the power to get at what’s extra elemental, resembling exit velocities and launch angles and the like — issues which might be in themselves extra predictive than their finish merchandise (the variety of homers). StatCast has its personal implementation of this type of train in its varied “x” stats. ZiPS makes use of barely totally different fashions with the same goal, which I’ve dubbed zStats. (I’m going to make you guess what the z stands for!) The variations within the fashions might be vital. For instance, when speaking about grounders, balls hit straight towards the second base bag turned singles 48.7% of the time from 2012 to ’19, with 51.0% outs and 0.2% doubles. But grounders hit 16 levels to the “left” of the bag solely turned hits 10.6% of the time over the identical stretch, and towards the second base aspect, it was 9.8%. ZiPS makes use of information like dash pace when calculating hitter BABIP, as a result of how briskly a participant is has an impact on BABIP and extra-base hits.
ZiPS doesn’t discard precise stats; the fashions all enhance from figuring out the precise numbers along with the zStats. You can learn extra on how zStats relate to precise stats right here. For these curious in regards to the r-squared values between zStats and actual stats for the offensive parts, it’s 0.59 for zBABIP, 0.86 for strikeouts, 0.83 for walks, and 0.78 for homers. Those relationships are what make these stats helpful for predicting the long run. If you possibly can clarify 78% of the variance in dwelling run price between hitters with no details about what number of homers they really hit, you’ve answered numerous the riddle. All of those numbers correlate higher than the precise numbers with future numbers, although a mannequin that makes use of each zStats and precise ones, as the complete mannequin of ZiPS does, is superior to both by themselves.
And why is that this vital and never simply number-spinning? Knowing that adjustments in stroll charges, dwelling run charges, and strikeout charges stabilized far faster than different stats was an vital step ahead in participant valuation. That’s one thing that’s helpful whether or not you’re employed for a entrance workplace, are a hardcore fan, need to make some fantasy league strikes, and even only a common fan who’s rooting in your faves. If we enhance our information of the fundamental molecular construction of a stroll or a strikeout, then we will discover gamers who’re bettering or struggling much more shortly, and supply higher solutions on why a stroll price or a strikeout price has modified. This is helpful information for me particularly as a result of I clearly do numerous work with projections, however I’m hoping any such data is fascinating to readers past that.
As with any mannequin, the proof of the pudding is within the consuming, and there are all the time some those who query the worth of information resembling these. So for this run, I’m pitting zStats in opposition to the final two months and all new information that clearly couldn’t have been used within the mannequin and not using a time machine to see how the zStats did in comparison with actuality. I’m not going to do an entire put up for this each time, however that is one thing that, based mostly on the suggestions from the final put up in June, folks actually wished to see the outcomes for.
I addressed hitters earlier this week. Today, we’ll flip our consideration to the pitchers. Starting with zBABIP, let’s take a look at how the numbers have shaken out for the leaders and trailers from again in June. I didn’t embrace gamers who confronted fewer than 100 batters over the past two months.
Home Run Overachievers (As of 6/1)
Home Run Underachievers (As of 6/1)
zHR was nearer on 16 of 21 overachievers, with a imply absolute error (MAE) of 0.9% vs. 2.1% for the precise dwelling run price. This is form of to be anticipated since zHR goes up in opposition to an especially risky stat — zHR higher have a decrease error price, even with the outcomes themselves being risky and in a fairly small pattern dimension. We solely have 13 of the underachievers returning with sufficient enjoying time, because of pitchers with excessive dwelling runs allowed regularly both being injured or shortly dropping their jobs. ZiPS was nearer on eight of the 13 (MAE 2.0% vs. 2.9%). For all pitchers, not simply leaders and trailers, ZiPS had an MAE of 1.4% in comparison with 2.4% for precise dwelling run price.
Walk Overachievers (As of 6/1)
Name | BB | zBB | zBB Diff | BB% (6/1) | zBB% (6/1) | BB% Since |
---|---|---|---|---|---|---|
Framber Valdez | 15 | 24.8 | -9.8 | 5.2% | 8.7% | 7.0% |
Zack Wheeler | 15 | 24.4 | -9.4 | 5.5% | 8.9% | 3.4% |
Tyler Wells | 12 | 20.7 | -8.7 | 5.0% | 8.6% | 9.9% |
Anthony DeSclafani | 9 | 16.9 | -7.9 | 3.3% | 6.2% | 7.5% |
Cristian Javier | 16 | 23.6 | -7.6 | 6.4% | 9.4% | 10.0% |
JP Sears | 11 | 17.0 | -6.0 | 4.5% | 7.0% | 6.4% |
Tanner Bibee | 10 | 15.8 | -5.8 | 6.3% | 10.0% | 9.0% |
Dean Kremer | 17 | 22.6 | -5.6 | 6.7% | 8.9% | 7.9% |
Zac Gallen | 16 | 21.4 | -5.4 | 5.5% | 7.4% | 4.9% |
Nathan Eovaldi | 14 | 19.4 | -5.4 | 4.9% | 6.8% | 10.3% |
Matt Brash | 9 | 14.3 | -5.3 | 8.7% | 13.8% | 10.7% |
Jesús Luzardo | 20 | 25.2 | -5.2 | 7.1% | 8.9% | 6.5% |
J.P. France | 8 | 12.9 | -4.9 | 6.8% | 11.0% | 7.6% |
Wade Miley | 9 | 13.9 | -4.9 | 5.3% | 8.1% | 10.6% |
Jordan Montgomery | 17 | 21.6 | -4.6 | 6.3% | 8.0% | 7.2% |
Taj Bradley | 5 | 9.6 | -4.6 | 4.1% | 7.9% | 10.1% |
Zach Eflin | 8 | 12.5 | -4.5 | 3.4% | 5.4% | 3.3% |
Josh Winckowski | 7 | 11.5 | -4.5 | 5.1% | 8.4% | 8.7% |
Yennier Cano | 1 | 5.5 | -4.5 | 1.0% | 5.5% | 7.3% |
Bryce Miller | 3 | 7.3 | -4.3 | 2.2% | 5.4% | 6.7% |
Walk Underachievers (As of 6/1)
zBB beat precise stroll price on 14 of the 20 returning overachievers and 14 of the 18 underachievers. The MAEs for the zStats had been each decrease as properly (1.9% vs. 2.9% and a couple of.4% vs. 3.3%). The identical sample held true for all pitchers, at 2.1% vs. 3.1%.
Strikeout Overachievers (As of 6/1)
Name | SO | zSO | zSO Diff | SO% (6/1) | zSO% (6/1) | SO% Since |
---|---|---|---|---|---|---|
Kevin Gausman | 100 | 79.9 | 20.1 | 32.9% | 26.3% | 33.7% |
Mitch Keller | 93 | 78.6 | 14.4 | 30.4% | 25.7% | 19.7% |
Merrill Kelly | 69 | 55.6 | 13.4 | 27.4% | 22.1% | 24.0% |
Logan Gilbert | 73 | 59.9 | 13.1 | 28.7% | 23.6% | 22.0% |
Zac Gallen | 82 | 69.5 | 12.5 | 28.4% | 24.1% | 24.4% |
Framber Valdez | 77 | 64.6 | 12.4 | 26.9% | 22.6% | 23.4% |
Andrew Heaney | 55 | 42.7 | 12.3 | 24.3% | 18.9% | 24.9% |
Taj Bradley | 42 | 31.0 | 11.0 | 34.4% | 25.4% | 27.4% |
Alexis Díaz | 41 | 30.7 | 10.3 | 48.8% | 36.6% | 25.8% |
Joe Ryan | 76 | 66.0 | 10.0 | 29.1% | 25.3% | 29.0% |
Clarke Schmidt | 65 | 55.0 | 10.0 | 26.0% | 22.0% | 18.4% |
Edward Cabrera | 66 | 56.1 | 9.9 | 28.4% | 24.2% | 25.7% |
Seth Lugo | 38 | 29.1 | 8.9 | 21.3% | 16.3% | 25.1% |
Yennier Cano | 30 | 21.2 | 8.8 | 30.0% | 21.2% | 18.3% |
Lance Lynn | 76 | 67.3 | 8.7 | 25.1% | 22.2% | 28.7% |
Kyle Freeland | 43 | 34.3 | 8.7 | 16.1% | 12.8% | 13.1% |
Grayson Rodriguez | 56 | 47.4 | 8.6 | 26.5% | 22.5% | 21.2% |
Hunter Brown | 74 | 65.6 | 8.4 | 28.8% | 25.5% | 24.0% |
Gerrit Cole | 79 | 70.9 | 8.1 | 26.2% | 23.6% | 27.3% |
Strikeout Underachievers (As of 6/1)
Name | SO | zSO | zSO Diff | SO% (6/1) | zSO% (6/1) | SO% Since |
---|---|---|---|---|---|---|
Shane Bieber | 53 | 70.4 | -17.4 | 16.9% | 22.4% | 24.0% |
Tyler Anderson | 33 | 48.8 | -15.8 | 13.8% | 20.4% | 23.3% |
Patrick Corbin | 42 | 56.8 | -14.8 | 14.0% | 18.9% | 16.5% |
Patrick Sandoval | 36 | 49.2 | -13.2 | 15.1% | 20.6% | 23.4% |
Johan Oviedo | 53 | 64.5 | -11.5 | 19.8% | 24.1% | 21.4% |
Shane McClanahan | 82 | 92.9 | -10.9 | 29.1% | 32.9% | 20.9% |
Nick Martinez | 37 | 47.8 | -10.8 | 19.4% | 25.0% | 24.2% |
Ian Gibaut | 22 | 31.3 | -9.3 | 20.6% | 29.3% | 20.3% |
Jon Gray | 46 | 54.6 | -8.6 | 19.9% | 23.6% | 20.7% |
Gregory Santos | 26 | 34.1 | -8.1 | 21.3% | 27.9% | 25.2% |
Sandy Alcantara | 60 | 68.0 | -8.0 | 20.5% | 23.2% | 20.1% |
Emmanuel Clase | 20 | 28.0 | -8.0 | 17.7% | 24.8% | 27.2% |
Corbin Burnes | 59 | 66.8 | -7.8 | 22.3% | 25.2% | 27.4% |
J.P. France | 26 | 33.4 | -7.4 | 22.2% | 28.6% | 15.2% |
For the overachievers, zSO was nearer to outcomes than the precise strikeouts had been for 13 of the 19 gamers (3.7% vs. 5.4%). I used to be involved about underachievers, provided that numerous the pitchers concerned had harm questions, however ZiPS nonetheless eked out wins right here each in roughly being nearer (eight of 14) and MAE (4.4% vs. 4.9%). For all pitchers, the MAE was 3.9% vs. 5.1%. Since I’ve been a very long time Kevin Gausman obsessee, I’m blissful to have zSO miss on that one.
FIP Overachievers (As of 6/1)
Name | FIP ER | zFIP ER | zFIP ER Diff | FIP (6/1) | zFIP (6/1) | FIP Since |
---|---|---|---|---|---|---|
Zac Gallen | 16.9 | 27.1 | -10.3 | 2.09 | 3.36 | 4.18 |
Sonny Gray | 14.7 | 23.4 | -8.6 | 2.20 | 3.49 | 3.31 |
Framber Valdez | 23.5 | 31.0 | -7.5 | 2.94 | 3.88 | 3.68 |
Kevin Gausman | 19.8 | 26.3 | -6.4 | 2.38 | 3.15 | 3.08 |
Bailey Ober | 14.5 | 20.4 | -5.8 | 3.24 | 4.54 | 4.22 |
Brad Hand | 4.5 | 10.0 | -5.4 | 2.01 | 4.42 | 5.95 |
Nathan Eovaldi | 21.0 | 26.4 | -5.4 | 2.54 | 3.19 | 4.32 |
Bryce Miller | 10.8 | 16.2 | -5.3 | 2.71 | 4.04 | 4.88 |
Yennier Cano | 4.6 | 9.9 | -5.3 | 1.43 | 3.06 | 4.30 |
Mitch Keller | 23.7 | 28.8 | -5.1 | 2.86 | 3.48 | 5.11 |
Chris Stratton | 8.9 | 13.6 | -4.7 | 2.58 | 3.94 | 3.34 |
Michael Wacha | 22.6 | 27.2 | -4.6 | 3.55 | 4.27 | 4.12 |
Bryce Elder | 25.0 | 29.0 | -3.9 | 3.43 | 3.97 | 5.38 |
Adam Wainwright | 13.1 | 16.9 | -3.8 | 4.47 | 5.77 | 6.52 |
Dane Dunning | 15.1 | 18.8 | -3.7 | 2.83 | 3.53 | 4.89 |
Fernando Cruz | 4.9 | 8.5 | -3.6 | 2.84 | 4.89 | 3.17 |
Cristian Javier | 27.0 | 30.6 | -3.5 | 3.82 | 4.32 | 5.41 |
Logan Allen | 13.0 | 16.3 | -3.4 | 2.94 | 3.71 | 4.83 |
Merrill Kelly | 24.2 | 27.6 | -3.4 | 3.42 | 3.90 | 4.57 |
Andrew Wantz | 7.9 | 11.1 | -3.2 | 3.29 | 4.62 | 9.27 |
Dauri Moreta | 8.4 | 11.6 | -3.2 | 3.01 | 4.16 | 3.61 |
Josh Hader | 5.8 | 9.0 | -3.2 | 2.35 | 3.63 | 2.24 |
Mark Leiter Jr. | 8.6 | 11.8 | -3.1 | 3.42 | 4.67 | 3.38 |
Emilio Pagan | 8.1 | 11.2 | -3.1 | 3.04 | 4.20 | 3.97 |
FIP Underachievers (As of 6/1)
Name | FIP ER | zFIP ER | zFIP ER Diff | FIP (6/1) | zFIP (6/1) | FIP Since |
---|---|---|---|---|---|---|
Chris Bassitt | 38.5 | 32.1 | 6.4 | 5.22 | 4.35 | 4.01 |
Corbin Burnes | 32.2 | 25.1 | 7.1 | 4.55 | 3.54 | 3.32 |
Taijuan Walker | 34.4 | 28.4 | 6.0 | 5.40 | 4.46 | 3.86 |
Patrick Corbin | 37.3 | 30.8 | 6.5 | 4.96 | 4.09 | 5.45 |
Aaron Nola | 36.5 | 29.8 | 6.7 | 4.40 | 3.59 | 4.23 |
Lance Lynn | 39.4 | 33.7 | 5.8 | 5.27 | 4.50 | 5.32 |
Yusei Kikuchi | 37.4 | 28.6 | 8.8 | 5.97 | 4.57 | 3.30 |
Colin Rea | 25.3 | 19.4 | 5.9 | 5.37 | 4.12 | 4.89 |
Luke Weaver | 26.2 | 20.0 | 6.2 | 5.40 | 4.12 | 6.21 |
Jon Gray | 29.7 | 23.4 | 6.3 | 4.64 | 3.65 | 3.91 |
Tyler Anderson | 31.4 | 24.1 | 7.3 | 5.36 | 4.12 | 3.39 |
Martín Pérez | 34.0 | 25.4 | 8.6 | 5.01 | 3.74 | 5.65 |
Ken Waldichuk | 39.5 | 31.0 | 8.5 | 7.02 | 5.51 | 3.50 |
Shane Bieber | 34.5 | 28.3 | 6.2 | 4.14 | 3.40 | 4.13 |
Julio Urías | 32.6 | 25.7 | 7.0 | 5.31 | 4.17 | 3.19 |
Ross Stripling | 24.1 | 17.9 | 6.3 | 6.72 | 4.98 | 3.64 |
Alek Manoah | 40.2 | 31.5 | 8.6 | 6.27 | 4.92 | 6.08 |
This is the place we tie every thing collectively, and it’s not a straightforward matchup contemplating one of many advantages of FIP is meant to be its lack of volatility. zFIP was nearer on 21 of 24 overachievers (MAE of 1.04 vs. 1.61) and on 9 of 17 underachievers (MAE of 1.01 vs. 1.23). For all pitchers, the MAE was 1.03 for zFIP, 1.52 for FIP).
On a year-to-year foundation, taking a look at gamers with 200 TBF in consecutive seasons (about 1500 gamers), ZiPS edges out the actual stats in all 4 classes. The MAE edges are 0.9% vs. 1.1% in homer price, 1.6% vs. 1.8% in strikeout price, 3.3% vs. 3.5% in stroll price, and 0.72 in opposition to 0.82 in FIP. Naturally, zStats mixed with the precise ones does even higher than both class individually. Based on historical past, I modeled the perfect linear mixture of zStats and precise numbers in predicting subsequent 12 months’s stats. For homers, it’s 92% zStats vs. 8% precise. While which may appear stunning, it’s merely as a result of the truth that dwelling runs for pitchers are extremely risky, which is why xFIP improves on FIP simply by assuming that homers are given up at a league-average price by all pitchers, an deliberately preposterous situation. For walks, it’s 72% zBB vs. precise. For strikeouts, the tally is available in at 57% zSO, 43% precise. As one closing reminder, zStats include no ingredient of regression towards the imply; it solely evaluates the Statcast/plate self-discipline information for the time intervals in query.
Tomorrow, I’ll have the up-to-date zStat leaderboards for the pitchers.
Content Source: blogs.fangraphs.com